One of the most significant issues of our day is climate change, which calls for swift and proactive responses from all facets of society. Artificial intelligence (AI) has become a key instrument in environmental monitoring and climate modeling, providing previously unheard-of capacity to evaluate enormous datasets and extract useful information.
The fight against climate change is becoming increasingly dependent on new technology, especially artificial intelligence (AI). In this chapter, the fundamental function of AI in comprehending and addressing ecosystem health and climate change is introduced. It is crucial to comprehend how AI and climate science interact to create strategies that effectively address environmental issues and advance sustainability.
Climate Modeling and AI’s Role
Climate modeling involves a lot of variables and can become complicated, much like attempting to solve a Rubik’s cube while wearing a blindfold. Fortunately, AI can help us sort through that chaos. Climate scientists can more thoroughly examine intricate relationships within the climate system by using AI. Predictive analytics and pattern recognition techniques are used by AI systems to find trends and abnormalities that human analysts might miss.
Climate scientists, data engineers, and AI experts must work together because AI in climate modeling is an interdisciplinary field. Effective AI-driven climate solutions necessitate a thorough comprehension of machine learning methodologies as well as environmental processes. The most common AI model types in studies about climate change are machine learning and deep learning.
* Deep learning: A form of machine learning known as “deep learning” analyzes several aspects of the climate system using multi-layered neural networks, hence the term “deep.” Unstructured data, like text and photos, are common in climate studies. For example, satellite images are used to follow storm tracks or land-use changes. These models are very good at processing these types of data.
* Machine learning: These models use past data to learn and generate predictions or judgments without the need for explicit programming. Based on weather data, machine learning can be used in climate science to forecast weather occurrences, forecast solar and wind power generation, and optimize energy-efficient technologies.
Finally, by offering tools that improve real-time responsiveness, operational efficiency, and prediction accuracy, artificial intelligence (AI) significantly advances climate modeling and environmental monitoring. Setting the scene for a thorough examination of how AI technology might be used to address the urgent problems of environmental degradation and climate change, this chapter provides background information.
Climate Prediction Using Machine Learning Algorithms
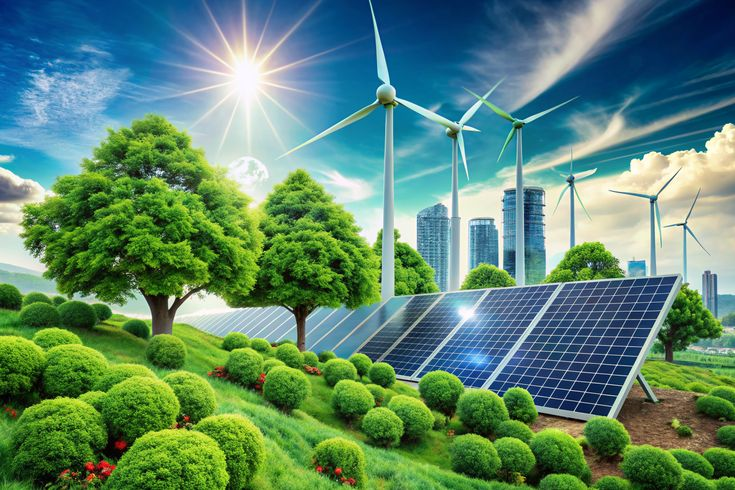
The computational capacity and analytical skills required to handle and interpret complex climate data are provided by machine learning (ML) algorithms, which are the foundation of AI-driven climate prediction models. Because they can simulate the relationships between input variables and desired results, supervised learning algorithms like Linear Regression, Support Vector Machines (SVM), and Neural Networks are frequently employed in climate prediction.
For instance, by recognizing intricate patterns and dependencies that conventional models could miss, neural networks can uncover nonlinear interactions in climate data, allowing for more precise forecasts of extreme weather events.
Climate prediction has greatly improved because to Deep Learning (DL), a branch of machine learning (ML), which makes it possible to process enormous amounts of high-dimensional data. Recurrent neural networks (RNNs) and convolutional neural networks (CNNs) are very good at interpreting climate data over time and space, respectively. By improving climate prediction systems’ capacity to recognize complex trends and produce more accurate long-term projections, these DL models help create more dependable climate resilience plans.
An increasingly potent tool in climate modeling is reinforcement learning (RL), which makes it possible to create adaptive models that can maximize decision-making in response to shifting climatic conditions. RL, for example, can maximize efficiency and reduce carbon emissions by optimizing the deployment of renewable energy resources based on real-time weather data. This flexibility is necessary to develop climate models that not only forecast future events but also guide preventative actions to lessen negative climate effects.
Using AI to Reduce Climate Change
According to action understanding, the management of renewable energy is arguably where AI’s contribution to climate mitigation is most evident. When it comes to improving the efficiency of power networks that incorporate various renewable energy sources, artificial intelligence algorithms are at the forefront.
Reducing pollutants from industrial processes and transportation is another crucial area where AI has a big impact on the sector. Applications of AI in transportation include controlling traffic patterns in cities to cut down on idle time and optimizing routes for electric cars to save energy. To cut down on downtime and energy loss, smart factories, for example, employ AI models to forecast equipment faults and optimize maintenance schedules.
Forecasting Environmental Trends with Predictive Analytics
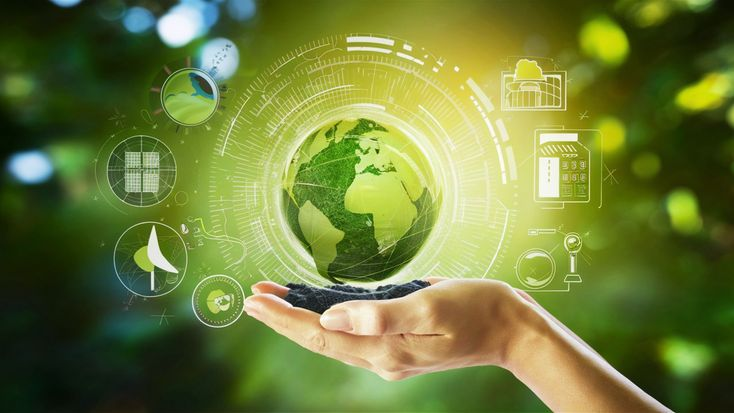
To anticipate environmental trends and take preventative action against climate change and ecosystem degradation, predictive analytics—powered by artificial intelligence (AI)—is essential. Organizations may make well-informed decisions and develop strategic plans by using machine learning models to forecast trends like temperature swings, precipitation patterns, and ecological changes.
Finding odd or unexpected changes in the environment that could indicate new problems is made possible by AI-driven anomaly detection, another crucial component of predictive analytics. AI systems can identify anomalies like abrupt temperature increases, unforeseen shifts in the health of the vegetation, or erratic water quality levels by examining departures from accepted standards.
The practical usefulness of AI-generated projections is increased when predictive analytics is integrated with decision support systems, offering policymakers and environmental managers useful information. Predictive models are used in decision support systems to model diverse situations and evaluate the possible results of various policy measures.
Predictive analytics, for example, can help create adaptive land-use plans that take into consideration anticipated shifts in ecosystem dynamics and climate, guaranteeing that agricultural practices and urban growth are in line with sustainability objectives.
AI for Adaptation to Climate Change
To effectively manage the effects of natural catastrophes like floods, storms, and droughts, AI improves disaster response and management by delivering precise and fast information. To improve preparedness and resource allocation, artificial intelligence (AI) models can, for example, reliably forecast the locations of extreme weather events by analyzing weather data, historical event data, and geographic information.
AI-enabled systems can forecast yields, track crop health, and even identify plant illnesses early on thanks to sophisticated images from satellites or drones. AI-powered robotic systems can also offer targeted pest management, which minimizes the environmental effect and eliminates the need for extensive pesticide use while increasing crop output.
AI’s Drawbacks and restrictions in climate modeling
Even though AI is revolutionizing climate modeling and weather forecasting, there are drawbacks. Let’s start by discussing data quality. The quality of data that is put into AI models determines their performance. Deep learning models in particular are examples of AI algorithms that function as “black boxes.”
This implies that even if they are capable of making precise forecasts, it can be challenging to comprehend how they did so. This lack of transparency can irritate climate scientists and meteorologists. If you ask a cat why it knocked over your beloved vase, you’re unlikely to get a satisfactory response.
Another issue is data privacy. Although most meteorological data is publicly available, there are ethical concerns when AI is combined with personal data (such as location-based services). How can we provide accurate forecasts without compromising individual privacy? Like walking a tightrope while balancing burning torches, it’s a delicate balance. And lastly, the problem of public opinion. People frequently misunderstand artificial intelligence and its potential. When projections don’t work out, some people may become disappointed because they believe AI can predict the weather with perfect accuracy. The potential advantages of AI in climate modeling and weather forecasting are enormous, notwithstanding these difficulties. Researchers can keep enhancing the precision and dependability of forecasts by tackling these constraints.
Future Prospects of AI in the Environment and Climate
Numerous new developments and trends are set to influence the future of AI in climate and environmental applications, increasing its potential and influence. The creation of autonomous and decentralized environmental monitoring networks will be made easier by the convergence of edge computing, IoT, and AI, which will improve the capacity for data gathering and processing.
The creation of AI-driven decision support tools that enable environmental managers and policymakers to make wise and efficient decisions is another important future path. AI-driven decision support systems improve an organization’s capacity to execute sustainable and strategic climate and environmental policies by offering thorough and actionable information.
Final Thoughts
One revolutionary tool in the fight against environmental degradation and climate change is artificial intelligence. Artificial Intelligence plays a crucial role in climate modeling, prediction, adaptation, and mitigation methods by improving our capacity to gather, evaluate, and act upon complicated climate data. However, AI is not a panacea, despite its potential. Ethical considerations about privacy and trust, interpretability problems, and data quality all limit its efficacy.
Transparent algorithm design, ongoing interdisciplinary cooperation, and inclusive public involvement will be necessary to overcome these obstacles. In the future, artificial intelligence (AI) combined with other cutting-edge technologies like robotics, edge computing, and the Internet of Things (IoT) portends a more intelligent, quick, and flexible approach to environmental stewardship.
Frequently Asked Questions (FAQs)
What part does AI play in managing renewable energy?
AI improves energy storage, forecasts renewable energy supply (such as wind and solar), and balances supply and demand on smart grids. Additionally, it can forecast equipment breakdowns, guaranteeing effective energy system maintenance.
Is it possible for AI to identify environmental changes that humans overlook?
Indeed, AI is excellent at spotting minute irregularities in huge datasets that could go unnoticed by humans. For instance, it can use sensor data and satellite photos to identify changes in biodiversity, pollution spikes, or early indicators of environmental stress.
In what specific ways does AI aid in the forecasting of extreme weather events?
AI can identify trends before catastrophic events like storms or heatwaves by analyzing both historical and current data. The ability to recognize temporal patterns that result in precise short- and long-term weather forecasts is a strength of deep learning models, particularly Recurrent Neural Networks (RNNs).