Drug discovery is the complex process of creating, identifying, and developing novel drugs to enhance human health and prevent illness. High expenses, protracted deadlines, and failure risk are just a few of the difficulties it presents. However, a revolutionary method of addressing these issues is provided by the development of artificial intelligence technology. Drug discovery has seen a dramatic change as a result of the application of AI.
AI has drastically changed the pharmaceutical sector by increasing drug discovery speed, increasing accuracy, and lowering costs. This blog is your comprehensive resource for learning about the advantages, common applications, difficulties, and practical applications of artificial intelligence (AI) in the pharmaceutical sector.
Using AI in Drug Discovery
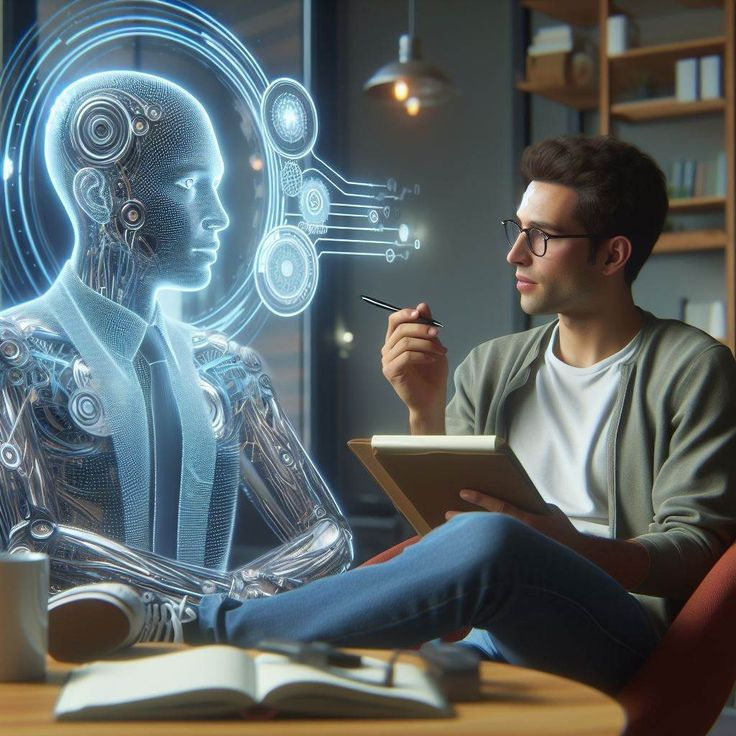
Different phases of the drug development pipeline are covered by AI applications. By utilizing artificial intelligence’s remarkable powers in data processing, pattern detection, and decision-making, the integration of AI technology has revolutionized pharmaceutical research. The many ways artificial intelligence is being used in the drug development process are the main topic of this section.
1. Forecast the Target Protein’s Three-D Structure
A crucial stage in structure-based drug design and discovery is the precise prediction of the target proteins’ three-dimensional (3D) structure. The collection of comprehensive protein sequence and structural data from many sources forms the basis of AI-driven protein structure prediction. With the use of this information, AI algorithms can be trained to recognize intricate patterns that connect amino acid sequences to their three-dimensional structures.
AI provides a quicker, more affordable option that generates dependable 3D models from sequence data. This development enables the creation of medications that are specific to the structure of the target protein, enabling early assessments of the safety and effectiveness of medications. Through graph machine learning approaches, AI has also demonstrated potential in modeling intricate relationships within biomedical data.
2. Chemical Synthesis with AI
In the field of drug discovery, the sustainability and efficiency of chemical synthesis are crucial. AI’s integration into chemical synthesis marks a significant advancement in drug development by increasing the accuracy and efficiency of synthetic procedures. This is achieved by combining automation, AI, and real-time reaction monitoring, which collectively allow experimental procedures to be significantly faster and more reliable. But there are drawbacks to depending too much on automated processes and AI algorithms, especially the possibility of oversimplifying the intrinsic complexity of chemical interactions.
3. Intelligent Cell Sorting Activated by Images
By detecting how cells react to medications, AI-powered imaging helps with high-throughput compound screening, improving target specificity, and facilitating the careful selection of promising therapeutic candidates. AI has previously been used for drug discovery by several healthcare organizations; the most well-known examples are provided in the section below.
4. Drug Development
AI greatly speeds up the drug development timeline in the field of drug design by improving the identification process of promising lead compounds. Finding tiny compounds that meet several essential requirements is the core of medication design.
A desirable safety profile, appropriate chemical and biological features, pharmacological efficacy, and the innovation required to protect intellectual property rights for economic viability are some of these. The goal of structural drug design is to find tiny compounds that can interact with protein targets in a specific way. This area of drug design has been completely transformed by the introduction of AI, especially deep learning and feature extraction techniques.
AI’s Advantages in current drug development
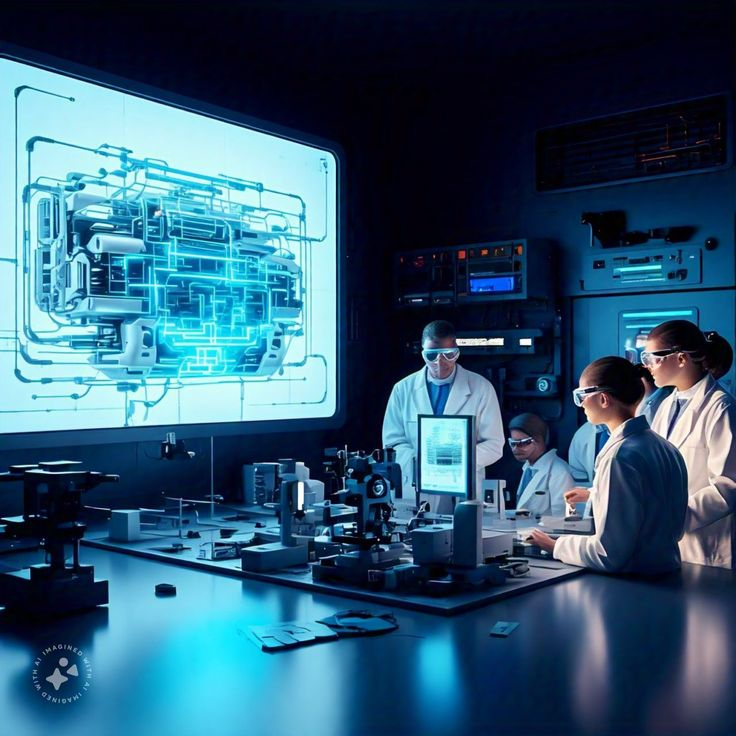
AI in drug discovery has several advantages, such as:
1. Wearable technology powered by AI
AI-powered wearables track patient reactions in real-time throughout clinical trials, offering vital information to enhance safety profiles and improve medication formulation. It also makes it possible to follow patients remotely, which lowers the need for frequent hospital stays and increases trial participation rates.
2. Improved Biomedical Data Interpretation
In drug discovery, artificial intelligence (AI) analyzes high-dimensional, complicated biological data to find trends and insights. It greatly improves the analysis of biomedical data and aids in the integration of data from various sources, such as clinical, proteomic, and genomic data, to provide a thorough understanding of disease causes.
3. Accelerated Drug Development
By automating procedures like molecular simulations and synthesis route generation, artificial intelligence (AI) helps to speed up the process, which is one of the main advantages of investing in this area of drug research and development activities.
4. Improved Analysis of Biomedical Data
AI in drug discovery analyzes high-dimensional, complex biological data to find trends and insights. A thorough grasp of disease mechanisms, it greatly improves biomedical data analysis and aids in the integration of data from many sources, such as genomic, proteomic, and clinical data.
Examples of AI in the Real World for Drug Discovery
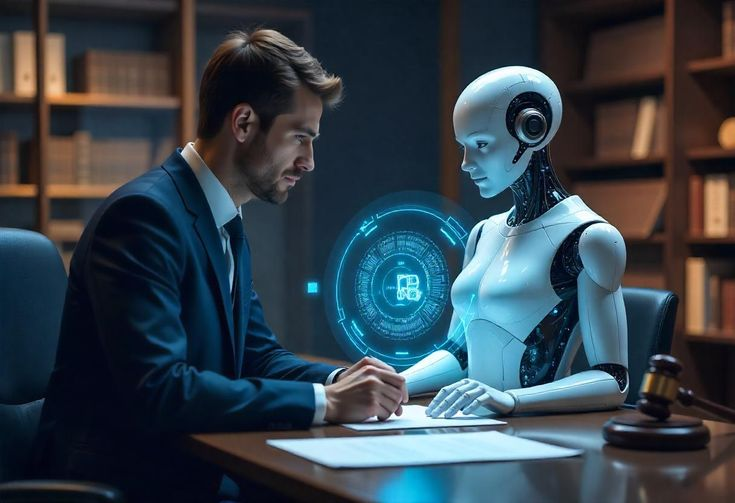
AI has already been used by several pharmaceutical companies to achieve the greatest outcomes. Here are some instances from the actual world.
1. The Healx
Healx is an AI company that specializes in treating rare diseases. The business modified its AI platform during the COVID-19 pandemic to find medications that were already on the market and could be repurposed to treat COVID-19 symptoms. Their method sped up the process of repurposing drugs and aided international research efforts by rapidly analyzing large datasets to find promising cures.
2. Atom-by-atom
Atomwise found AT-121, a possible medication for pain relief, using its AI-powered platform. By acting as a dual-target opioid receptor modulator, this substance reduces pain without having the same addictive potential as conventional opioids. The AI technology drastically shortened the time needed for discovery by screening millions of molecules in a matter of days. Preclinical research on AT-121 has yielded encouraging results, underscoring AI’s potential to create safer pain management options.
3. Recursion Drugs
Recursion Pharmaceuticals discovered REC-994, a therapy for Cerebral Cavernous Malformation (CCM), a rare vascular disease in the brain, using its AI-driven drug discovery platform. To find prospective therapeutic candidates, the platform examined biological data and cellular images. As an example of how AI might speed up the development of treatments for uncommon and complicated diseases, REC-994 is currently undergoing Phase II clinical trials.
Limitations and Difficulties in AI-Assisted Drug Development
The potential for creating materials with particular qualities for a variety of purposes is enormous when AI is used to help with material discovery. However, to guarantee its successful execution, some obstacles and restrictions must be recognized and addressed.
1. Availability and Quality of Data
For training, AI models need a lot of high-quality data. Because so many different materials and properties might be considered, it can be challenging to gather thorough and reliable datasets in materials research. Predictions that are biased or incorrect can arise from incomplete or wrong data.
2. Scalability of Artificial Intelligence Solutions
Even while AI is excellent at evaluating big datasets, it is still difficult to scale these models to effectively accommodate ever-increasing data volumes. For wider use, it is essential to provide computational resources and energy efficiency.
3. Selection of algorithms
The particular issue at hand will determine which AI algorithms are most suited for the finding of a substance. It takes knowledge of both AI and materials science to create specialized algorithms that can manage the particular difficulties of material data, such as high dimensionality and non-linear connections. Although AI models are remarkably accurate in predicting material qualities, it is still difficult to understand the underlying causes of these predictions. To direct future research and design, it is crucial to comprehend the connection between the input qualities and output properties.
Prospects for AI-Assisted Drug Discovery in the Future
Artificial intelligence (AI)-powered platforms are being used to virtually screen vast libraries of compounds, finding possible therapeutic candidates more accurately and effectively than with conventional techniques. To help choose the most promising candidates for additional research, these systems can also forecast the pharmacokinetic and toxicity profiles of lead compounds and optimize them.
Additionally, by making it possible to create new chemical structures with certain characteristics, AI is revolutionizing medication creation. The possibilities of AI also go beyond the research and discovery of new drugs. Additionally, it is essential for patient classification, clinical trials, and real-time therapy response monitoring, allowing for more individualized medical methods that improve patient outcomes.
In Conclusion
AI is transforming drug discovery in the pharmaceutical sector by improving cost-effectiveness, speed, and accuracy. Drug development is accelerated by AI at every stage, from predicting protein structures to optimizing chemical synthesis and evaluating intricate biology data.
AI’s practical application in the development of novel therapies is demonstrated by businesses such as Healx, Atomwise, and Recursion Pharmaceuticals. The future of AI-assisted drug development appears bright, despite obstacles like algorithm scalability and data quality. As AI develops further, it will spur innovation, enhance patient outcomes, and fill gaps in medicine, revolutionizing the search for and development of novel medications.
FAQs on AI in Drug Discovery
AI in drug discovery: what is it?
The term “artificial intelligence” in drug discovery describes the application of AI tools, such as machine learning and deep learning, to speed up and enhance the process of finding, creating, and refining new medications. It facilitates the analysis of huge datasets, molecular interaction prediction, and chemical compound optimization.
How is drug discovery enhanced by AI?
AI improves accuracy, efficiency, and cost-effectiveness in drug discovery. It speeds up processes like chemical synthesis, target identification, and protein structure prediction. It also makes it possible to screen drug candidates more quickly and analyze complex biomedical data more effectively.
What role does AI play in drug discovery going forward?
AI-powered drug research in the future will involve increasingly sophisticated algorithms for real-time patient monitoring, tailored therapy, and virtual screening. AI will continue to improve patient outcomes, increase efficiency, and meet unmet medical requirements as technology develops.