Numerous sectors have been revolutionized by artificial intelligence (AI), and the fiction industry is no exception. AI is completely changing the way we tell tales because of the most recent developments in machine learning and natural language processing.
AI-generated stories are raising the bar for what we previously believed was feasible because of their capacity to produce intricate narratives, compelling characters, and even emotional depth. As long as you are aware of its limitations, generative AI can help writers in many ways. The state-of-the-art AI storytelling methods, resources, and upcoming advancements influencing the fiction genre are explored in this extensive book.
The Development of Artificial Intelligence and the History of Storytelling
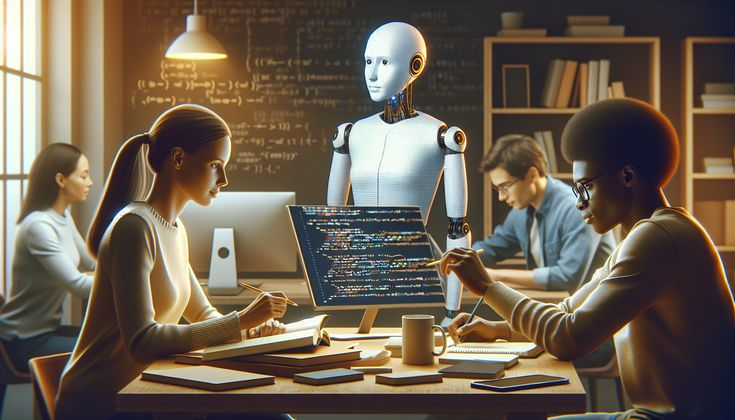
For thousands of years, storytelling has been an essential component of human culture. Throughout history, people have utilized stories to communicate their experiences, values, and ideas to one another, from the first cave paintings to the most recent blockbuster movies.
The emergence of AI is one of the most fascinating and recent advancements in narrative technology. Although artificial intelligence has been a popular term for a few years, its potential in narrative is still being explored.
Yet, compared to the history of narrative in general, the history of AI-generated storytelling is brief. Machines could only create straightforward stories with predictable results in the early days of artificial intelligence.
Although AI-generated narrative is still in its early stages, it has a lot of promise. The stories that robots can tell will only get more complex and captivating as they learn and develop. But the emergence of narratives produced by AI also poses significant queries regarding the place of people in the creative process.
Methods for Generating Stories Using AI
1. Rule-Based Frameworks
Earlier attempts in AI storytelling used rule-based systems, which created stories using preset templates and rules. These systems use a predetermined set of rules and logic to decide, carry out actions, or draw conclusions. Rule-based systems are still essential because of their interpretability, transparency, and ease of use, even in the face of more sophisticated AI techniques like machine learning and neural networks.
2. The use of neural networks in deep learning
Deep learning methods and neural networks have been the driving forces behind recent developments in AI storytelling. These models, like GPT-3, can produce more sophisticated and varied stories because they can learn patterns and structures from vast textual databases. For many issues in image identification, audio recognition, and natural language processing, neural networks, and deep learning now offer the best answers.
3. Symbolic Artificial Intelligence
To generate narratives, symbolic artificial intelligence, often known as GOFAI (Good Old-Fashioned AI), uses logic-based reasoning and symbolic representations of information. Algorithms using symbolic artificial intelligence process symbols, which stand in for real-world items or ideas, and their connections.
By representing and modifying knowledge and reasoning according to logical rules, symbolic AI aims to create intelligent systems that are capable of reasoning and thinking similarly to humans. These systems frequently use semantic networks to depict the links between tale elements and integrate writers’ expert knowledge.
AI-generated storytelling’s advantages
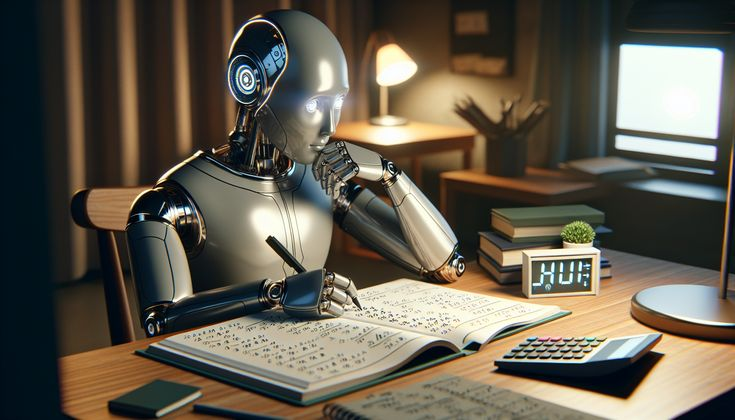
1. Efficiency
The capacity of AI-generated storytelling to produce stories rapidly and effectively is one of its main advantages. Machines are far more efficient than humans at processing large volumes of data and creating narratives. In fields where fresh information is constantly needed, like advertising or news, this may be immensely helpful.
2. Diverse
Machines can produce stories that reflect a greater variety of experiences and viewpoints since they are not subject to the same biases and preconceptions as people. This can result in a media environment that is more varied and inclusive.
Important AI Narrative Models
1. BERT
Google created a language model that has already been trained, called BERT (Bidirectional Encoder Representations from Transformers). BERT has been utilized in AI storytelling applications, despite being primarily created for problems involving natural language processing. BERT’s architecture is encoder-only. There are encoder and decoder modules in the original Transformer architecture. An encoder-only design is used in BERT, indicating that comprehension of input sequences is prioritized over production of output sequences.
2. GPT-3.
The cutting-edge language model GPT-3 (Generative Pre-trained Transformer 3) was created by OpenAI. GPT-3 can produce text that seems like it was written by a human, and it has been used to produce a variety of AI-generated writings, ranging from short stories to novels. GPT-3, Open AI’s replacement for their prior language model (LM), GPT-2, was unveiled earlier in May 2020. It is regarded as larger and superior to GPT-2.
3. Models of transformers
Transformer models have transformed AI storytelling and are the foundation of both GPT-3 and BERT. Their distinct design, which includes self-attention processes, enables them to produce more logical and contextually relevant stories by capturing long-range dependencies.
To identify subtle ways that even distant data pieces in a series influence and depend on one another, transformer models employ a developing collection of mathematical approaches known as attention or self-attention. By identifying patterns and irregularities, transformers can stop fraud, expedite production, provide online advice, or enhance healthcare.
AI-generated storytelling’s drawbacks include:
1. A lack of originality
The story’s lack of originality and emotional nuance is among the most significant. Machines are adept at adhering to systems and patterns, but they have trouble telling stories that are genuinely original and emotionally compelling. Stories that accurately depict the human experience still require human imagination and intuition.
2. The danger of telling stories
Even if robots are not as biased as people, they can nonetheless reinforce negative stereotypes and learn from biased data. As AI-generated storytelling proliferates, this is a significant ethical issue that requires attention.
Successful AI-generated narrative examples
Even while AI-generated stories are still in their infancy, there are already several effective applications of this technology.
One notable example is”1 the Road,” a book authored by Ross Goodwin’s AI system. In this experiment, Goodwin created text while traveling by car from New York to New Orleans in 2017 using a neural network. To record data in real-time, the AI was outfitted with several sensors, such as a clock, a microphone, and a GPS. This data passed into the AI, which produced a continual stream of writing. Even though the language was uncensored and fantastical, it captured the voyage in a distinctive, avant-garde manner, demonstrating how AI can support imaginative narrative.
The short science fiction movie “Sunspring,” which was created by researchers Ross Goodwin and Oscar Sharp and was written entirely by an AI dubbed Benjamin, is another example of a successful AI-generated story. The screenplay was created by the AI using a dataset of hundreds of science fiction scripts.
The film was made and acted by human actors, which brought the AI’s vision to life even though the speech and plot were frequently bizarre and abstract. “Sunspring” showcased the promise of artificial intelligence in creative writing and filmmaking when it debuted at the 2016 Sci-Fi London Film Festival.
Another illustration of artificial intelligence in the news sector is Reuters’ Lynx Insight, a tool driven by AI that helps reporters by sifting through massive datasets and spotting noteworthy patterns. In addition to providing ideas and insights for stories, it assists reporters in rapidly processing financial data, political developments, and other data.
Instead of taking the place of journalists, Lynx Insight improves their work by managing laborious data analysis so that they may concentrate on interpretation and narrative. News reporting has become faster and more accurate as a result of this partnership between AI and human reporters.
All things considered, these illustrations show how AI-generated storytelling can provide excellent and captivating stories in a range of settings. Even if this technology has some limitations, it is evident that it is a tool that is already having an impact on the fields of journalism, gaming, literature, and advertising.
In Conclusion
Across many industries, AI-generated storytelling is transforming the way narratives are produced. It creates complex narratives and a range of viewpoints by utilizing cutting-edge technology like deep learning and symbolic AI.
This innovation complements human ingenuity and increases efficiency, particularly in journalism and entertainment. AI storytelling has drawbacks, such as restricted creativity and possible biases, despite its benefits. AI’s increasing impact is demonstrated by successful instances like “1 the Road,” “Sunspring,” and Reuters’ Lynx Insight. AI will continue to influence storytelling as technology advances, presenting fresh possibilities while depending on human intuition for emotional nuance and originality.
FAQs on AI-Generated Storytelling
Can writers be replaced by AI?
No, AI helps human authors create material, but emotional intelligence and human creativity are still necessary for original stories.
In what ways does AI produce narratives?
Rule-based systems, deep learning neural networks, and symbolic AI are some of the techniques used by AI to create tales. These techniques examine vast amounts of textual data to create logical narratives.
Which AI-generated narratives have proven to be effective?
Reuters’ Lynx Insight for data-driven journalism, the AI-written science fiction movie “Sunspring,” and Ross Goodwin’s AI system’s “1 the Road” are a few.