The heavy manufacturing sector has costly and disruptive downtime. In sectors such as cement and steel, a single malfunction can interrupt production and result in large losses. By analyzing past equipment data, artificial intelligence (AI) plays a critical role in predictive maintenance by forecasting probable problems.
Predictive maintenance scheduling is made possible by AI, which reduces unscheduled downtime and prevents expensive production interruptions by recognizing patterns and early warning indicators. By 2033, the market for AI-driven predictive maintenance is projected to have grown from $723 million to $2,306 million, demonstrating the growing significance of AI-driven solutions in reducing downtime and streamlining operations.
Recognizing Artificial Intelligence
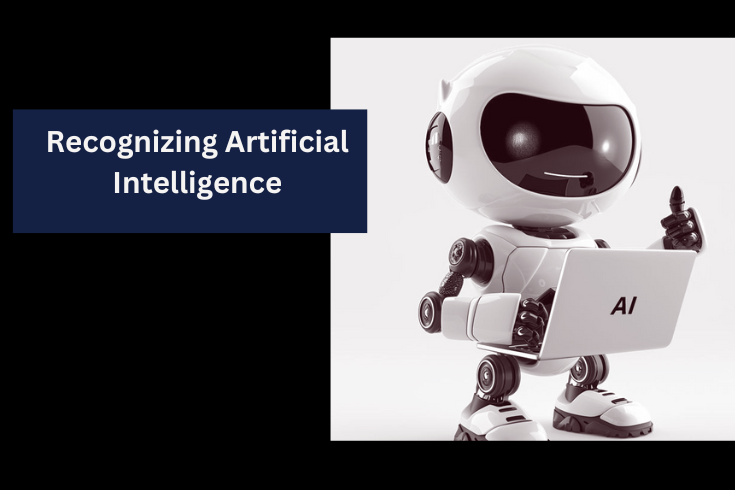
With the use of artificial intelligence (AI), robots can understand, learn, and solve problems by simulating human intelligence. AI examines enormous volumes of data in predictive maintenance, finding trends, and forecasting the performance and health of the equipment.
Traditional maintenance procedures become predictive rather than reactive due to AI’s capacity to proactively anticipate and handle maintenance requirements. This facilitates quicker and more effective decision-making, which eventually lowers downtime and boosts operational effectiveness.
Do you know what Predictive Maintenance is?
The revolutionary method of equipment maintenance known as Predictive Maintenance (PdM) aims to foresee and stop malfunctions before they happen. Utilizing cutting-edge technologies, it uses data to forecast any problems, enabling manufacturers to carry out maintenance only when required.
In addition to extending the lifespan of vital assets, this proactive approach avoids unscheduled downtime, maximizes machine usage, and lowers maintenance expenses. By predicting and preventing equipment failures, it allows you to prolong the life of machinery, drastically reduce downtime, and ultimately save money.
How Does AI Predictive Maintenance Operate?
Advanced computer vision techniques are used by artificial intelligence systems to gather and evaluate enormous volumes of data instantly. By enabling computers to comprehend and analyze visual data, these technologies aid in the early detection of mechanical wear.
A methodical methodology and several steps are involved, such as data gathering, processing, rule-making, analysis, and data-driven decision-making. The effectiveness of a factory predictive maintenance system powered by AI is fueled by the following essential elements.
1. AI Algorithm:
The foundation of AI and predictive maintenance is comprised of subsets such as machine learning and deep learning. They are essential in distinguishing data points and signals from noise and can be learned over time.
2. Sensors
In AI-based predictive maintenance, sensors are the key tools used to gather data on variables like vibration, pressure, and temperature. All of these real-time data points are connected to the performance and health of assets to create an analytical set.
3. Integrating and communicating:
The component makes sure that information moves smoothly throughout the company. Interaction and communication among many stakeholders, including experts, management, and maintenance staff, are crucial.
Major AI Uses in Predictive Maintenance
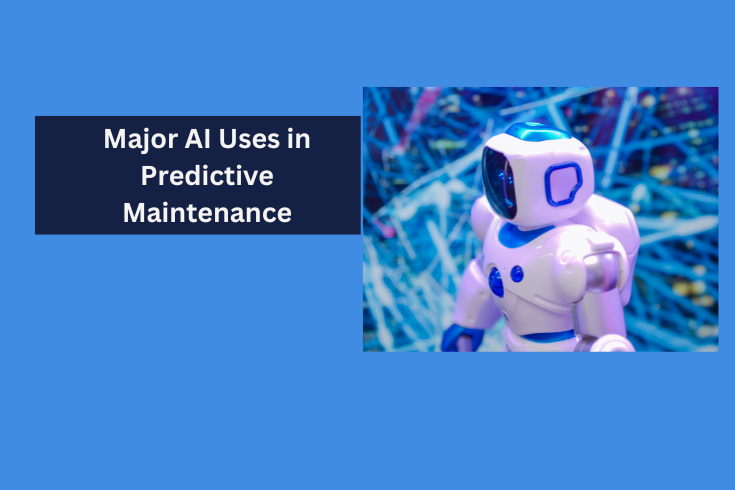
1. Anomaly Identification
AI systems can identify departures from standard operating parameters by analyzing real-time data from sensors installed in machines. A pump’s pressure falling below a given threshold or a motor vibrating more than normal are two examples of anomalies that AI can identify as possible early warning indicators of a malfunction.
2. Predictive analytics
AI can analyze past data to forecast when a certain piece of equipment is likely to break based on its performance history and present operating circumstances. As a result, there is less chance of unplanned malfunctions and less downtime since maintenance personnel can plan repairs or replacements at the best possible moments.
Important AI Uses for Predictive Maintenance
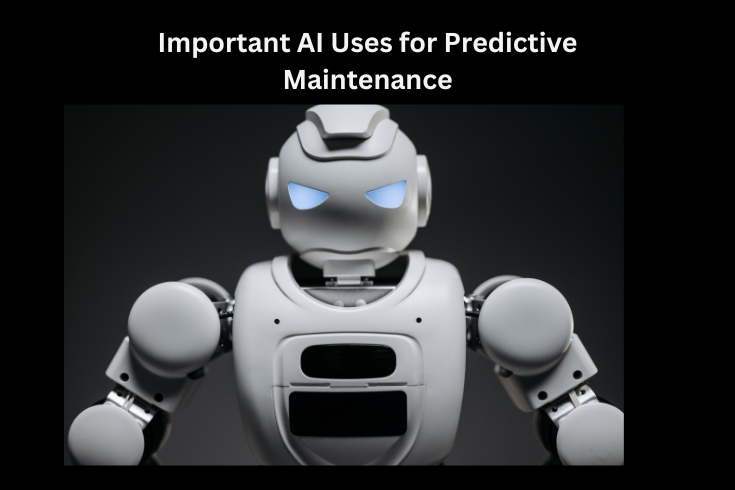
1. Finding Anomalies
Real-time data from sensors installed in machinery can be analyzed by AI algorithms to identify departures from standard operating parameters. AI can identify abnormalities as possible early warning indicators of a failure, such as when a pump’s pressure falls below a predetermined threshold or a motor begins to vibrate more than usual.
2. Analytics for Prediction
Based on its historical performance and present operating conditions, AI can analyze historical data to forecast when a certain piece of equipment is likely to break. This lowers the chance of unplanned malfunctions and minimizes downtime by enabling maintenance crews to plan repairs or replacements at the most convenient times.
3. Schedules of maintenance
Maintenance is typically carried out according to a set timetable, which may result in either too much or too little upkeep. However, AI-driven predictive maintenance makes sure that maintenance is only done when it’s required by customizing maintenance plans according to the actual state of the equipment. In addition to saving time and money, this prolongs the equipment’s life.
AI’s Advantages for Predictive Maintenance
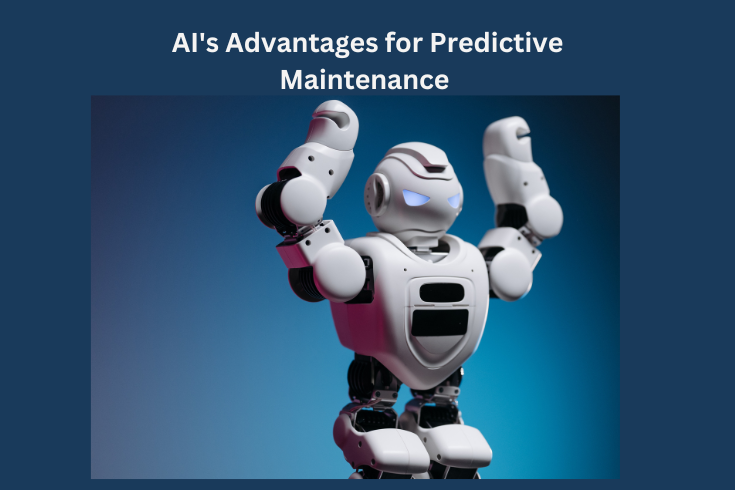
The use of AI in predictive maintenance has several benefits, including:
1. Less Idle Time:
Artificial intelligence can anticipate unexpected malfunctions before they occur, which reduces the need for unplanned equipment shutdowns. This guarantees continuous operations and eliminates needless downtime expenses, increasing overall productivity.
2. Making maintenance programs more efficient
Condition-based maintenance, in which repairs are made only when necessary, is made possible by AI in heavy industries. For instance, computer vision-driven systems in cement plants can track kiln bearing wear and arrange repair at the best moment, saving money on personnel and parts.
3. Extends Asset Lifespan:
AI can detect deterioration and match patterns when equipment and assets are in use, guaranteeing ideal results. Equipment can be kept in good working order for a longer amount of time by taking appropriate measures before a breakdown happens.
4. Durability
AI extends the life of equipment as well. Artificial Intelligence (AI) prolongs the life of machines by preventing excessive wear and tear and anticipating when maintenance is required. By doing this, the total return on investment (ROI) for current assets is increased while capital expenditures for new equipment are decreased.
Steps to Implement AI-Based Predictive Maintenance
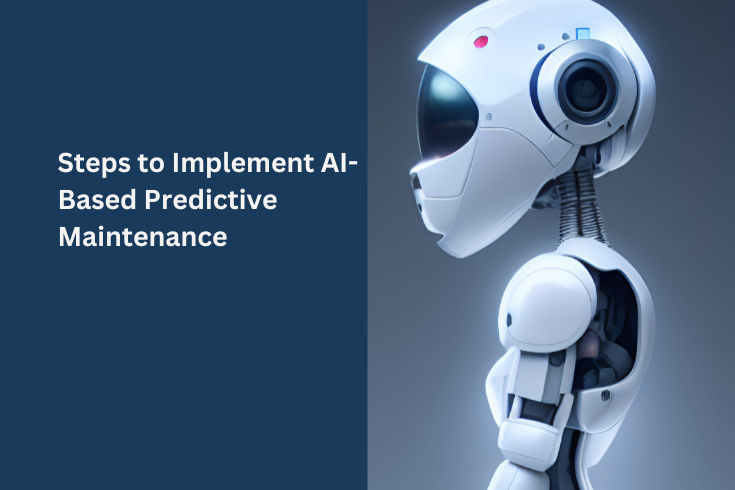
1. Define Clear Objectives:
Give the AI system measurable, precise goals to work toward, like reducing equipment downtime or improving maintenance scheduling. Well-defined goals offer an organized structure for execution and performance assessment.
2. Collect the Correct Data:
AI cannot function well without high-quality data. Gather data from operating systems, maintenance records, and sensors. Make sure all pertinent information on the operation of your equipment is included and that the data is accurate.
3. Preprocessing data
involves standardizing formats, eliminating or reducing data discrepancies, and dealing with empty values in the data set.
4. Create AI Models:
Choose and apply appropriate AI models, including artificial neural networks or machine learning techniques. To find trends and anticipate possible equipment breakdowns, train these models using past performance data.
5. Implement and Integrate:
After the AI models are prepared, put them into use in your operational setting. They ought to integrate easily with the maintenance and monitoring procedures you now use.
6. Monitor and Optimize
Keep an eye on the AI system’s performance at all times, make necessary adjustments to the models in response to user feedback, and change the system’s maintenance procedures to improve results.
Difficulties and Things to Think About
1. Data Availability & Quality
Making sure that data flows continuously from all of the important machinery is one of the main challenges. IoT sensors must be retrofitted into legacy devices, which might not have sensors built-in or produce little data, to reach the required degree of data comprehensiveness.
2. Price & Return on Investment
Several variables, such as the operation’s size, the importance of the equipment under observation, and the frequency of equipment breakdowns, affect return on investment (ROI). Businesses may need months or even years to fully reap the financial rewards of predictive maintenance, particularly if the initial investment is substantial.
3. Adjustment of the workforce
Predictive maintenance with AI necessitates a change in approach and expertise. Training maintenance personnel to use AI-driven tools and technologies may include learning new software, comprehending AI algorithms, and deciphering insights produced by AI. Lack of technological know-how and resistance to change can impede the use of AI in predictive maintenance.
Lastly,
Heavy industries are being revolutionized by AI-driven predictive maintenance, which lowers maintenance costs, increases productivity, and decreases downtime. Businesses may increase productivity and asset longevity by utilizing AI for real-time anomaly identification and predictive analytics.
Long-term gains are ensured by planned deployment, despite obstacles such labor adaption, data availability, and initial expenses. Businesses using predictive maintenance will have a competitive advantage and promote long-term success as AI use increases.
FAQ’S
How does AI vary from conventional upkeep techniques?
Conventional maintenance can be either preventive (planned at predetermined intervals) or reactive (fixed after failure). To anticipate faults and plan maintenance only when required, AI predictive maintenance is proactive and uses real-time data.
How will predictive maintenance powered by AI develop in the future?
As AI becomes more widely used, predictive maintenance will improve accuracy, automation, and cost-effectiveness, allowing industries to increase output while reducing downtime.
How will predictive maintenance powered by AI develop in the future?
As AI becomes more widely used, predictive maintenance will improve accuracy, automation, and cost-effectiveness, allowing industries to increase output while reducing downtime.