Imagine obtaining a diagnosis from an algorithm for a medical condition without knowing the rationale, or having a loan declined by an AI system without knowing why. These situations demonstrate why, in our increasingly AI-driven world, explainable AI (XAI) has become essential. A black box model is one in which the inputs and outputs may be seen, but the decision-making or internal mechanisms are hidden and difficult for people to comprehend.
Despite their potential for great accuracy and efficiency, black box models are frequently criticized for being challenging to audit, analyze, and explain. Decisions made in industries like healthcare, finance, or law enforcement can have a big impact, therefore this is especially troubling.
Explainable AI: What is it?
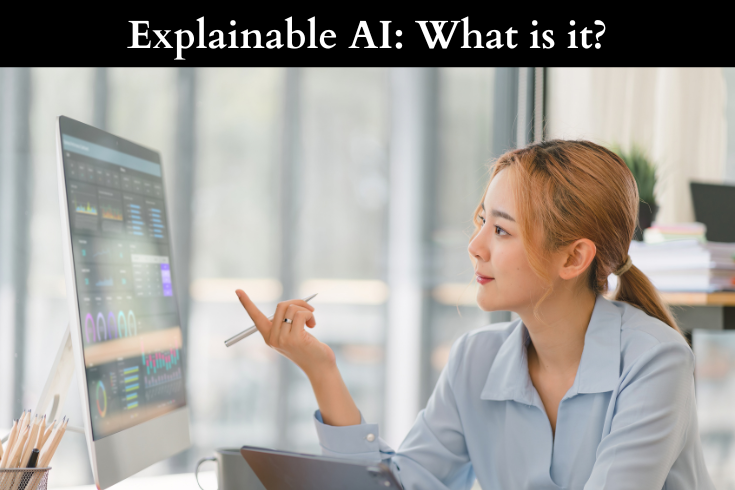
A collection of procedures and techniques known as explainable artificial intelligence (XAI) enables human users to understand and have faith in the output and outcomes produced by machine learning algorithms. When implementing AI models in production, a company needs explainable AI to foster confidence and trust.
An organization can also embrace a responsible approach to AI development with the aid of AI explainability. Explainability may be required to comply with regulatory requirements, assist developers in making sure the system is operating as intended, or be crucial in enabling anyone impacted by a decision to contest or alter the result.
What makes Explainable AI significant?
Virtually everything on the internet, from music suggestions to house assistants, is rapidly relying on machine learning algorithms. Explainable AI is becoming increasingly important for AI systems because it encourages research into creating more compelling justifications for the choices AI systems make.
The implementation of responsible AI, a paradigm for the widespread use of AI techniques in actual businesses with fairness, model explainability, and accountability, depends in significant part on explainable AI. Additionally, explainable AI supports productive AI use, model auditability, and end-user trust. Additionally, it reduces the risks of producing AI’s security, legality, compliance, and reputation.
Explainable AI is becoming increasingly important for AI systems because it encourages research into creating more compelling justifications for the choices AI systems make. Therefore, explainable AI offers us a chance to create new, useful technologies and assist us in improving AI systems in the future.
Why does Explainable AI exist?
The goal of XAI is to increase AI’s interpretability and transparency for people, especially in situations requiring sophisticated decision-making. The reason that many AI models are still regarded as “black boxes” is that they are difficult for humans to comprehend and analyze. Understanding the AI system’s decision-making process is crucial for many real-world applications.
XAI systems therefore seek to provide human-understandable justifications for AI judgments. Model visualization, feature importance analysis, and the creation of natural language explanations are some methods that can do this.
Real-World Examples of Explainable AI
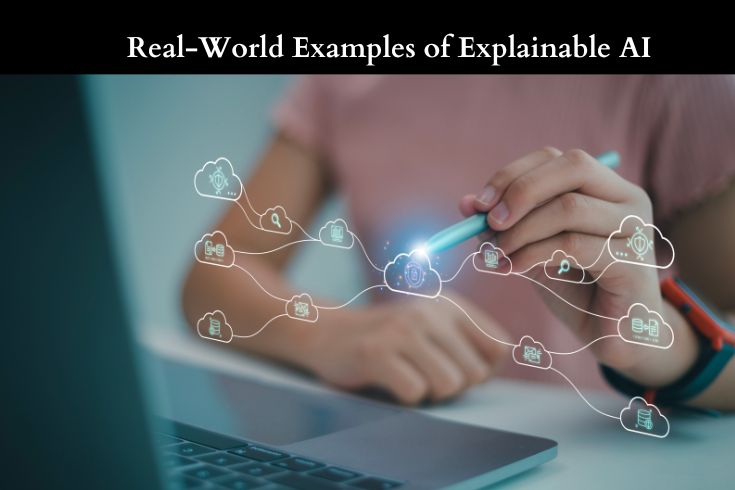
It alludes to open and understandable machine learning models that show how they arrived at their conclusions. Here are some instances of its use:
Legal Document Analysis: AI models help attorneys spot important terms, possible hazards, and compliance problems in contracts and other legal documents. They aid legal experts in making well-informed conclusions by offering justifications for their evaluations.
Fraud Detection: To identify fraudulent transactions, financial institutions employ AI algorithms. Investigators can make better conclusions by using its models to comprehend the reasoning behind the model’s decision to mark a certain transaction as fraudulent.
Client Support Chatbots: AI-driven chatbots help clients by giving answers based on past exchanges and corporate guidelines. Additionally, they can explain a particular response, guaranteeing openness in customer service.
Healthcare therapy Suggestions: AI models make tailored therapy recommendations for individuals based on their test findings, medical history, and present health issues. By explaining the rationale behind a prescribed treatment, they assist physicians in making more educated choices.
Important Elements of Explainable AI
Knowing how AI systems make judgments is essential for establishing confidence as AI gets more and more integrated into our daily lives.
1. Interpretability: Understanding AI Choices
Interpretability enables us to comprehend the rationale behind particular choices. This element guarantees that both technical specialists and regular consumers can comprehend AI results. When a system has good interpretability, it may point to particular symptoms or test findings that support its conclusions and explain its diagnosis in a way that both patients and medical professionals can understand. The ability of interpretable AI to deconstruct complicated judgments into manageable chunks is what makes it so beautiful.
2. Transparency in Models: Penetrating the Dark Box
Consider transparency in AI as being similar to gazing through a window made of clear glass rather than a dark box. This entails disclosing how an AI system was developed, what data it was trained on, and how it makes judgments, per IBM’s research on AI transparency.
Documenting the AI’s reasoning and disclosing the data sources that were utilized to train the system are further aspects of transparency. Transparent AI systems offer a clear understanding of their decision-making processes, just like we expect human decision-makers to explain their thinking.
3. Accountability: Making Certain AI Is Responsible
Accountability, which identifies who is responsible for AI decisions and their effects, is the last component. This element ensures that there are methods in place to recognize and address biased results or errors made by AI systems. Having appropriate governance and monitoring systems in place is another aspect of accountability. Regular audits of AI systems, explicit protocols for handling issues, and assigned teams in charge of keeping an eye on and upholding AI equity are a few examples of this.
Explainable AI’s advantages
1. Put AI into practice with confidence and trust.
Develop confidence in AI creation. Get your AI models into production quickly. Make sure AI models are understandable and interpretable. Increase the transparency and traceability of the model while streamlining the evaluation process.
2. Improved decision-making:
By providing people with information about how the AI model arrived at its conclusion, XAI can assist humans in making better decisions. It can assist humans in identifying and fixing any biases or mistakes in the model.
3. Reduce the expense and danger of model governance
Make sure your AI models are transparent and comprehensible. Control risk, compliance, regulations, and other requirements. Reduce costly mistakes and the overhead of human examination. Reduce the possibility of inadvertent prejudice.
All things considered, XAI can contribute to the transparency, interpretability, and reliability of AI, which will increase its usefulness and effectiveness across a range of applications.
Explainable AI’s Disadvantages
There are still a lot of open problems with Explainable models in this relatively new field of study.
1. Complexity vs. Interpretability:
Deep learning and other advanced AI models are extremely complicated and frequently operate as “black boxes,” making it challenging to provide a straightforward explanation of their decision-making procedures.
2. Lifecycle automation:
Create, execute, and oversee models as a component of AI and data integration services. To track models and exchange results, consolidate the tools and procedures into a single platform. Describe how machine learning models are dependent on one another.
3. Trade-off The Relationship Between Explainability and Accuracy:
Complex models like neural networks are frequently more accurate than more interpretable models like decision trees. It can be difficult to strike a balance between transparency and performance.
Final Thoughts
Explainable artificial intelligence (XAI) improves accountability, transparency, and trust in AI-driven choices in sectors like healthcare, finance, and law. By making AI models easier to understand, it aids businesses in reducing risks, adhering to regulations, and making better decisions. Nonetheless, difficulties still exist, such as striking a balance between interpretability and complexity and guaranteeing accuracy while preserving fairness.
XAI developments will allow for the responsible application of AI, guaranteeing that its judgments are fair and intelligible despite these obstacles. Explainability should be given top priority so that companies and society may fully utilize AI’s potential while promoting ethical AI development and trust for a more transparent future.
FAQs on Explainable AI (XAI)
How can businesses benefit from XAI?
XAI increases decision-making, lowers compliance risks, boosts customer trust, and makes sure AI systems adhere to legal and ethical requirements.
What prospects does Explainable AI have?
For the ethical deployment of AI, the future of XAI entails creating more interpretable models, enhancing legal frameworks, and incorporating AI transparency into commonplace applications.
What effects does XAI have on AI ethics?
By encouraging equity, responsibility, and openness, XAI ensures responsible AI development and aids in detecting biases in AI models.