AI Bias : Our everyday lives now depend on AI since it makes a wide range of applications and technology possible. However, prejudice has become a problem as AI systems develop further. Both the model’s design and the training data it uses are common causes of bias in AI algorithms.
Models can favor particular results because they occasionally reflect the assumptions of the developers who created them. Several stages in the AI process, such as data collecting, algorithm design, and system implementation, are susceptible to bias. There are several reasons for this, such as ingrained biases in the data, historical injustices, or societal assumptions. The origins of AI bias, its practical manifestations, and the importance of tackling it will all be covered in detail in this article.
What does AI bias mean?
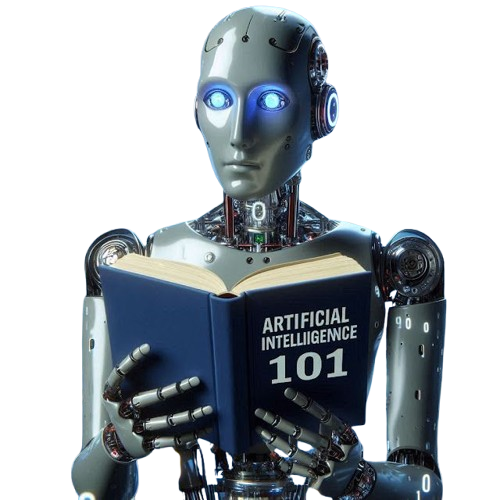
One definition of bias in AI is an inaccuracy that results in unfair decisions. Data collection, algorithm design, human biases, and other factors can all contribute to this, which is also known as algorithmic bias or machine learning bias. It is essential to comprehend bias in AI due to the potential repercussions for both individuals and society.
When biased AI systems reward or discourage individuals based on factors like gender, age, ethnicity, or socioeconomic status, they might reinforce discrimination. This may lead to disparate chances and worsen already-existing social injustices. Fairness and justice principles may also be compromised by biased AI systems that result in unfair decisions in critical areas including criminal justice, loan approvals, and hiring.
Importance of addressing AI bias
Humans are inherently biased. It results from a narrow view of the world and the propensity to generalize knowledge to expedite learning. But when biases hurt other people, ethical problems occur. Human-biased AI technologies have the potential to systematically increase this harm, particularly if they are incorporated into the institutions and frameworks that govern our contemporary lives.
Think of e-commerce chatbots, healthcare diagnostics, human resources hiring, and law enforcement monitoring. All of these tools have the potential to increase productivity and offer creative solutions, but if not used properly, they also come with serious risks. These AI technologies’ biases have the potential to worsen already-existing disparities and give rise to brand-new kinds of discrimination.
Discriminatory results can also result from biases in generative AI solutions. When creating job descriptions, for instance, an AI model must be built to prevent unintentionally omitting particular groups or using biased wording. Ignoring these biases may result in unfair hiring practices and the continuation of employment inequality.
By identifying strategies to reduce prejudice before utilizing AI to inform decisions that impact actual people, examples such as these highlight the importance of responsible AI practice for enterprises. To protect people and uphold public confidence, AI systems must be made fair, accurate, and transparent.
AI Bias Types
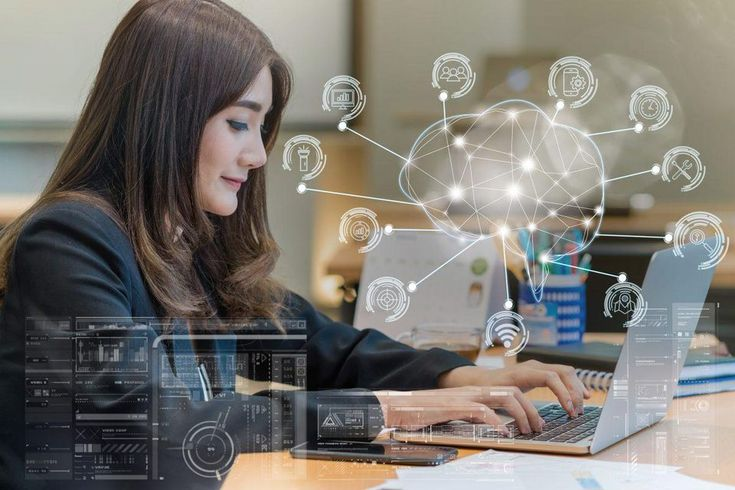
The following lists the several kinds of biases that exist in artificial intelligence.
1. Sampling Bias:
This bias results in poor performance and unfair, biased conclusions when the training dataset’s sample is not representative of the entire population it serves. As we previously saw, this kind of bias can arise in situations such as facial recognition systems, which perform poorly on persons of color and those with darker skin tones if the system is trained mostly on white individuals.
2. Algorithmic Bias:
This bias results from errors in the algorithm’s implementation and design. This kind of bias occurs when the system favors particular characteristics over others, resulting in unjust decisions. This bias, which is a systemic error, can be repeated and can be brought on by inadequate algorithm design or a lack of input data.
3. Confirmation Bias:
This is the phenomenon whereby the system draws conclusions based on preexisting prejudices held by the users or system programmers. This kind of bias prevents the system from recognizing new patterns in the data and restricts it to the historical trends in the data.
4. Measurement Bias:
This bias arises when data measures some groups and either overrepresents or underrepresents them. This can also happen when different groups’ accuracy varies. This kind of bias can mostly arise when surveys are being collected, with a primary focus on metropolitan areas that underrepresent rural areas.
5. Generative Bias:
The bias that arises in the generative AI model is known as generative bias. Using a variety of inputs, the Generative AI model generates new data, including texts and images. Unbalanced representations in the content of the model output result in this kind of bias. The resulting data is biased and unjust as a result of this prejudice.
6. Reporting Bias:
This bias arises when the training dataset’s event frequency and the actual frequency of events do not coincide. Sentiment analysis models exhibit this bias, as the training dataset might not fairly represent the sentiment distributions. This may also occur when a restaurant or product is reviewed using the system and a disproportionately high number of good evaluations outnumbers negative reviews, creating a skewed perception of the sentiment.
7. Automation Bias:
This bias arises when automated systems are given preference over non-automated methods, even when error rates are taken into account. The primary cause of this prejudice is people’s propensity to trust technology because they believe it to be automation systems are more efficient.
8. Group attribution prejudice
is a bias that has a tendency to assume that people have the same ideas as the group they are a part of. According to this presumption, if a person is a member of a group, they will likely share traits and make comparable choices.
Bias in AI examples
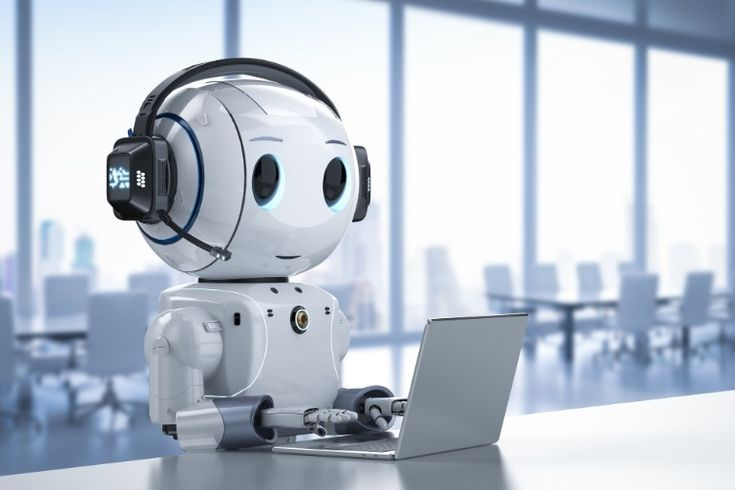
Bias in AI has the potential to have significant and pervasive effects on many facets of society and people’s lives.
Examples of how bias in AI might affect various situations include the following:
1. Hiring and recruitment: Prejudices in the workplace may be reinforced via screening algorithms and job description generators. Women and caregivers may be impacted by a technology that penalizes employment gaps or favors traditional words associated with men.
2. Education: Algorithms used for admission and evaluation may be biased. An AI that forecasts student achievement, for example, would give preference to students from wealthy schools over those from underfunded ones.
3. Facial recognition: AI algorithms frequently have trouble accurately identifying demographics. For example, they may make more mistakes when identifying darker skin tones.
4. Social media and content moderation: Policies may not always be applied uniformly by moderation algorithms. For instance, compared to members from the majority group, posts from minority users may be unjustly classified as offensive.
5. Healthcare: Biases in diagnosis and treatment recommendations may be introduced by AI. Systems that have been trained on data from one ethnic group, for instance, may misdiagnose other ethnicities.
6. Voice recognition: Conversational AI programs may exhibit prejudice against particular dialects or accents. For instance, regional accents or non-native speakers may be difficult for AI assistants to understand, which would decrease their usefulness.
What effects does AI bias have?
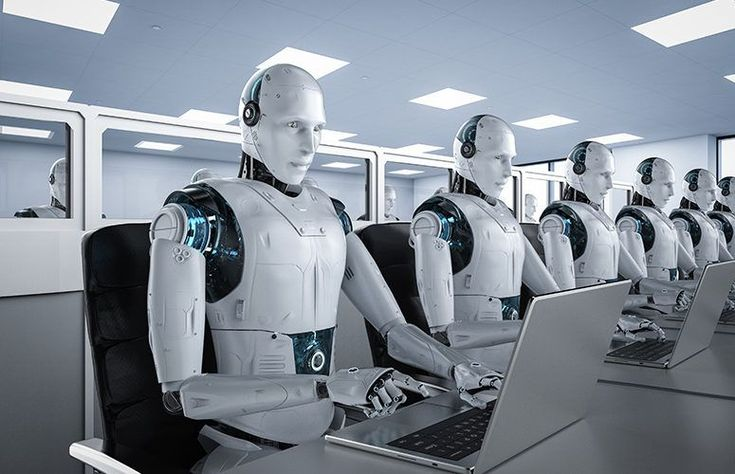
Economic effects:
Certain groups may be unjustly disadvantaged by biased algorithms, which could restrict employment possibilities and maintain inequality in the workplace. Certain populations may receive worse service from AI-driven customer support platforms, such as chatbots, which could result in discontent and lost revenue.
Stereotype reinforcement:
Harmful stereotypes can be strengthened by biased AI systems, which can sustain unfavorable opinions and treatment of particular groups on the basis of gender, race, or other traits. Gender bias is perpetuated, for instance, when natural language processing (NLP) algorithms link particular occupations to a particular gender.
Unfair Decision-Making:
In important spheres of life, including criminal justice or healthcare, biased AI systems may lead to unfair conclusions. For instance, people may be unfairly profiled or given harsher sentences based on their background rather than the specifics of their case if biased algorithms are employed to predict recidivism probabilities.
Discrimination
AI systems that are biased may continue to discriminate against individuals based on their gender, age, ethnicity, or socioeconomic status. Biased AI systems have the potential to worsen social injustices and keep some groups out of decision-making processes like loan approvals and employment. This kind of prejudice compromises the ideas of fairness, equality of treatment, and equal opportunity.
Bias in Artificial Intelligence: Difficulties
The following are the difficulties posed by bias in artificial intelligence:
The Algorithmic Bias
Algorithmic bias is another significant challenge that arises during the design and deployment of AI systems. Inadvertently amplifying or adding additional biases to the data is possible if algorithms are not carefully planned and assessed. To combat algorithmic bias, AI systems must be ready to be transparent, accountable, and egalitarian.
Assessing and Auditing
Assessing and keeping an eye on AI systems for bias is challenging. It is crucial to routinely evaluate AI systems for biased findings because the propensity can develop or change. Frequent audits and evaluations help spot biases early on and address them, promoting accountability and ongoing advancement in AI technologies.
Ethics-Related Issues
Resolving prejudice in AI requires tackling difficult moral dilemmas. Ethical norms and standards are essential to ensure fairness, transparency, and accountability in artificial intelligence systems. The development and responsible application of AI systems depend on the establishment of explicit ethical frameworks that place a high priority on bias prevention.
In conclusion
Artificial intelligence bias is a serious issue that needs to be addressed right now to stop it from hurting people and perpetuating social injustices. Although AI systems have enormous potential to boost creativity and productivity, abuse or poor design can have discriminatory effects.
To combat bias, equitable algorithms must be developed, representative datasets must be produced, and accountability, justice, and openness must be upheld. Businesses and governments can guarantee AI serves everyone without sustaining injustice or prejudice by implementing responsible AI policies.
FAQ’S
How can businesses deal with prejudice in AI?
- Employing representative and varied datasets is one strategy that organizations can use.
- carrying out bias audits regularly.
- integrating fairness metrics into the creation of AI.
- educating staff members on moral AI practices.
- ensuring AI systems are accountable and transparent.
Is it possible to completely eradicate prejudice in AI?
Even though it might not be possible to completely eradicate bias, it can be greatly decreased by employing a variety of datasets, carefully crafting algorithms, and conducting frequent audits and assessments.
Why is it crucial to overcome AI bias?
To maintain justice, respect moral principles, and stop prejudice, it is imperative to address AI bias. Unchecked bias can exacerbate societal inequality by resulting in unequal treatment in crucial domains such as criminal justice, healthcare, and employment.