Humans lived without the internet in what seems like a very long time ago in a galaxy far, far away. The internet went from being a technology used in science fiction to a commonplace one with over 5 billion users globally in a matter of decades. Since generative AI has been made widely available by recent technology advancements, its popularity is expected to grow more quickly than that of the World Wide Web. Nowadays, generative AI is pervasive. But what is it specifically? How is it operated? How can we utilize it to simplify our lives and work?
Generative AI is only going to grow in popularity as we move into a new era of artificial intelligence.
This article will go into great detail regarding generative AI and examine two of its most well-known uses: DALL-E and ChatGPT. We’ll explain these technologies, their functions, and the effects they’re having on different sectors of the economy.
Why is generative artificial intelligence currently a hot topic?
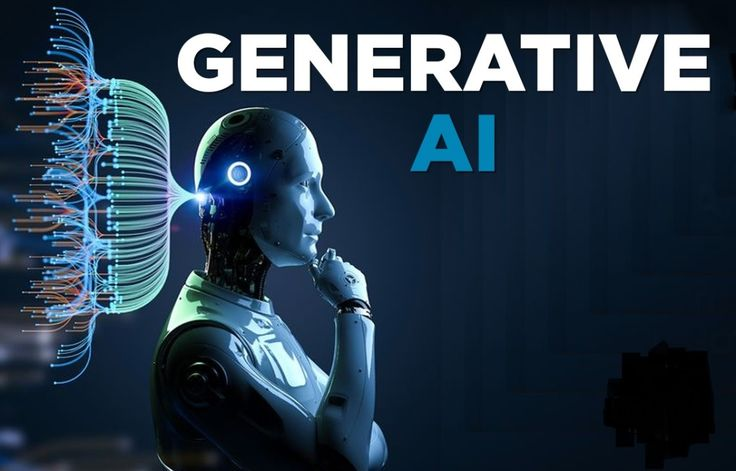
In recent years, generative AI has advanced significantly. One of the leading companies in the industry and the quickest AI product to reach 100 million users is ChatGPT, which you have most likely already used. Other well-known and up-and-coming AI technologies like DALL-E, Bard, Jasper, and others have people talking. Large tech firms are competing with startups to use the potential of AI applications, whether that means changing search engine optimization, achieving substantial market capitalization, or innovating in other areas.
Generative AI: What Is It?
A revolutionary kind of artificial intelligence called generative AI is revolutionizing the way that robots produce content. It is a branch of artificial intelligence that focuses on producing new data—text, photos, or other types of information—that is similar to the original data it was trained on.
Fundamental Ideas:
1. Deep Learning: This technique uses multi-layered deep neural networks to identify intricate patterns in data.
2. Neural Networks: Computational models based on the human brain that process information in layers using interconnected nodes (neurons).
3. Unsupervised Learning: Generative models frequently use unsupervised learning, which teaches them the underlying structure of input data without the need for explicit labels.
What is the process of generative AI?
Generative AI analyzes massive data sets using machine learning (ML) methods. This implies that artificial intelligence can be trained to recognize patterns and structures by being fed a large amount of existing data about a subject. Generative AI can produce fresh, unique content based on the knowledge it gains from this data.
A generative AI model trained on numerous cat photos, for example, might produce a new image that resembles a cat. Alternatively, a model that has been exposed to a large number of text descriptions may produce a new paragraph about a cat that sounds as though it was written by a human. The created material is new pieces that suit the patterns the AI has identified rather than exact replicas of what it has previously seen.
The same is true with generative AI. Learning from several examples is the first step. These could be text, music, pictures, or other types of information. By examining these instances, the AI gains knowledge of the structures and patterns that are there. It can begin to produce new examples that resemble what it has previously seen after it has gained sufficient knowledge.
Generative AI’s History
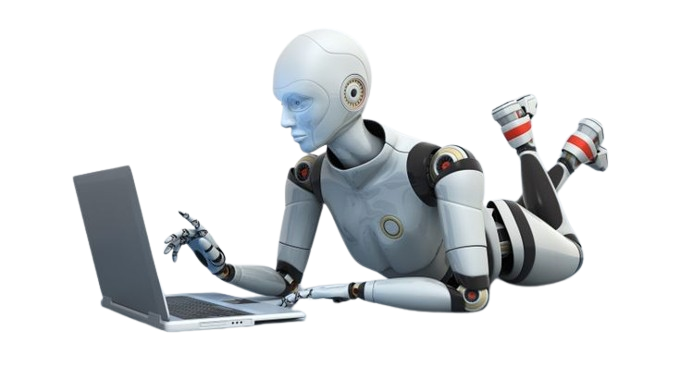
Early examples of generative AI, such as the ELIZA chatbot, were first seen in the 1960s. ELIZA created what appeared to be unique responses by simulating conversations with people. The chatbot’s powers were constrained, though, because these answers were dependent on a rules-based lookup database.
The 2014 launch of Generative Adversarial Networks (GANs) by Google researcher Ian Goodfellow marked a significant advancement in the field of generative artificial intelligence. A discriminator, a generator, and two networks are used in GANs, a kind of neural network design. While the discriminator assesses the newly produced content against a dataset of actual examples, the generator produces new material.
Another important development occurred in 2017 when a team at Google published the well-known Transformers article, “Attention Is All You Need.” In this instance, “attention” refers to systems that offer context according to a text’s word placement, which can differ between languages.
The researchers suggested ignoring other methods of extracting patterns from text in favor of concentrating on specific attention mechanisms. With transformers, it became possible to analyze a string of text all at once rather than processing it word by word, enabling significantly larger models.
Generative AI Types
Different generative AI model types exist, each with its methodology:
Variational Autoencoders (VAEs): VAEs decode input data back to the original data domain after encoding it into a latent space. In contrast to conventional autoencoders, VAEs sample from the latent space to produce new data by imposing a probabilistic framework on it.
Generative Adversarial Networks (GANs): GANs are made up of two neural networks that compete with one another: a discriminator and a generator. The discriminator assesses the new data instances that are produced by the generator. The generator’s objective is to mislead the discriminator by producing data that is identical to genuine data.
Transformer-based Models: Transformer-based models, such as GPT, process input data using self-attention techniques, which enables them to identify long-range dependencies in sequences. In text generation, where coherence and context are essential, these models work very well.
Diffusion models: These models reverse a diffusion process by iteratively transforming noise into a structured output. These models are becoming more popular in industries where fine detail is essential because they are known to provide high-quality photographs.
What are Bard, DALL-E, and ChatGPT?
For the general audience, DALL-E, ChatGPT, and Bard are among of the most popular, widely used, and potent generative AI tools.
ChatGPT
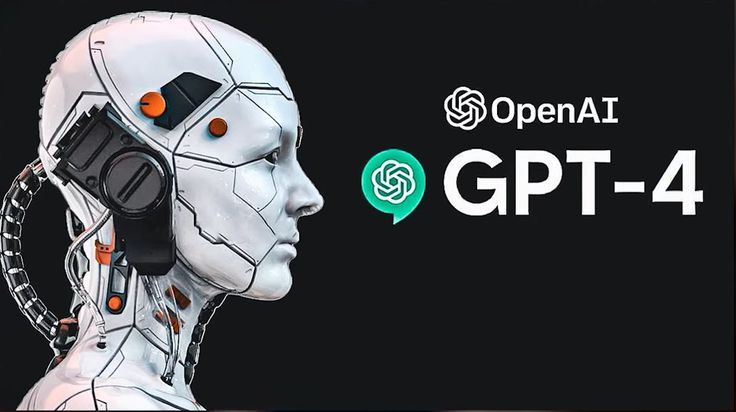
OpenAI created a language model called ChatGPT. Its foundation is the Generative Pre-trained Transformer (GPT) design, which is among the most sophisticated transformers on the market right now. With the ability to respond to a variety of prompts and queries conversationally, ChatGPT is intended to connect with users.
How ChatGPT’s Works?
ChatGPT’s primary method of processing and producing text is deep learning.
Transformer Architecture: ChatGPT is built on the Transformer model, which processes incoming text concurrently using self-attention methods to capture context and intricate dependencies.
Pre-training: The model is trained to predict the subsequent word in a sequence using a sizable dataset of online text. This enables it to comprehend and produce material that is coherent across a wide range of themes.
Fine-tuning: Following pre-training, ChatGPT is adjusted based on user feedback to enhance its conversational skills on certain tasks or datasets.
Contextual Understanding: By keeping context throughout a conversation, ChatGPT facilitates more organic and cohesive discourse.
Dall-E
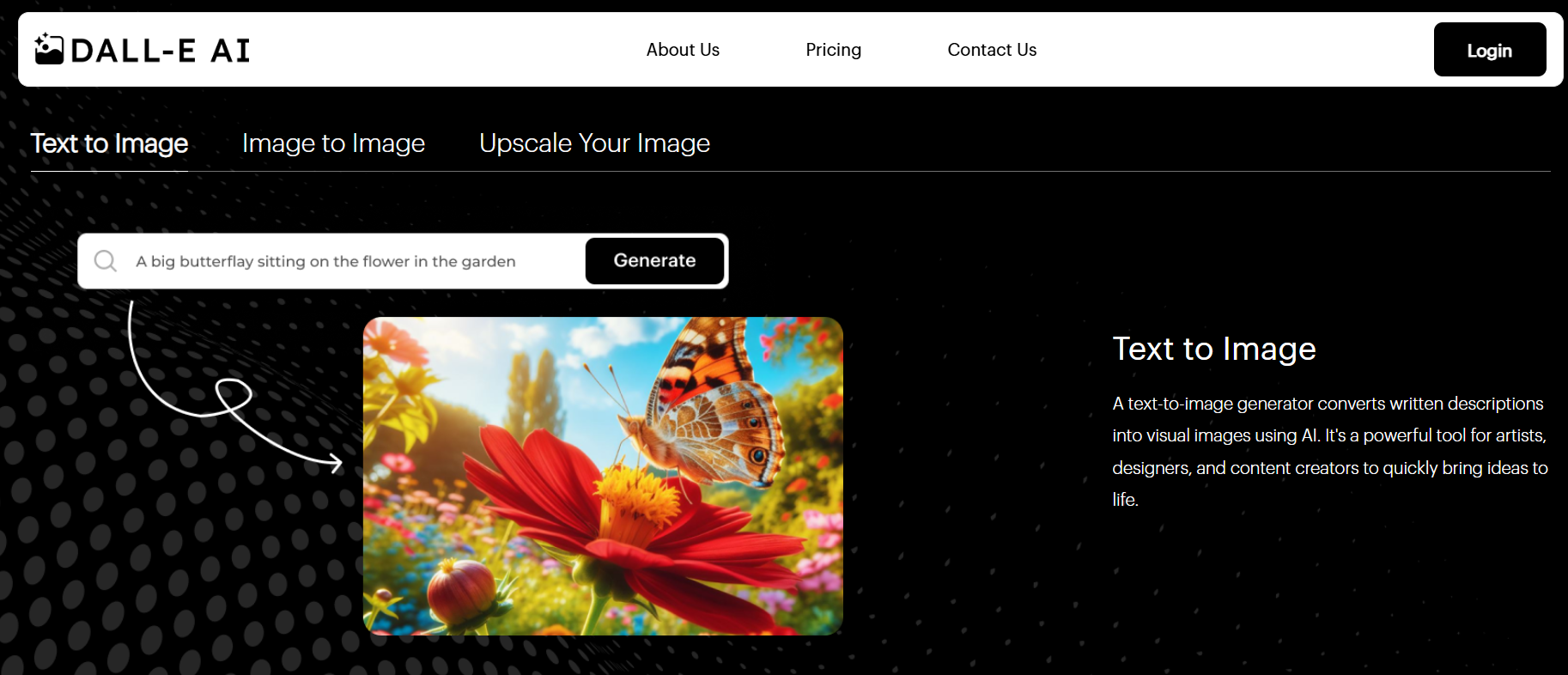
DALL-E is an artificial intelligence model that uses textual descriptions to create unique visuals. Based on textual prompts, DALL-E generates images completely from scratch, in contrast to conventional image creation algorithms that alter preexisting images. The model is trained using a combination of supervised and unsupervised learning methods on a large dataset of text-image pairs.
How Does It Work?
The process by which the input text is converted into a sequence of embeddings that capture the semantic meaning of the description serves as the foundation for the development of images.
Image Decoder: After learning patterns during training, the image decoder uses the encoded text to create images by forecasting pixel values or patches.
Variational Autoencoders (VAE): DALL-E uses VAEs to guarantee that the generated images are not only high-quality but also coherent and closely aligned with the input text.
Training Process: To learn how to map written descriptions to related images, the model is trained using a sizable dataset of image-text pairs. It can now generalize and produce new images in response to invisible cues thanks to this training.
Text encoder: Creates the foundation for image production by converting the input text into a sequence of embeddings that capture the semantic meaning of the description.
Bard
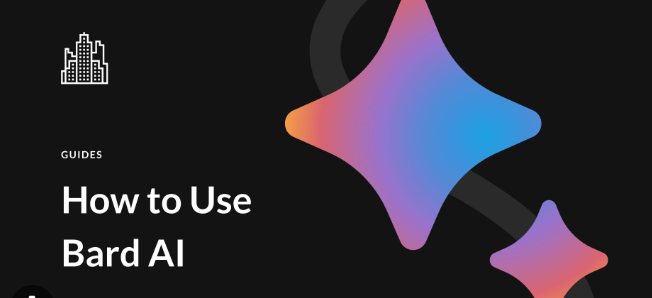
Google’s foray into the AI chatbot space is called Bard Bard. Google provided open-source research for others to build upon, making it an early pioneer in AI language processing. Because Bard is based on Google’s most sophisticated LLM, PaLM2, it can produce multimodal content—including real-time images—quickly.
How does it operate?
Bard’s backend is built on top of the PaLM2 language paradigm. The Pathways language model 2, which was introduced in 2022, is another name for it. In addition to PaLM2, Bard makes use of LaMDA technology, which Google introduced in 2017. These technologies are utilized in numerous additional AI-based products, such as ChatGPT’s GPT-3 model.
Because the backend is comparable, Bard uses its natural language processing capabilities to take your questions and offer the most helpful and natural response. Additionally, Bard accepts more than just keywords; you can enter full questions to receive the finest and most natural-sounding response.
It is made feasible by the fact that Bard’s backends’ artificial intelligence has been trained on millions of naturally sounding requests, questions, and answers. The fact that Bard follows up on the questions is something that further enhances it. It recognizes the kinds of queries you have been posing and responds with pertinent information to any follow-up queries.
Lastly, some thoughts
The quick development of generative AI tools like ChatGPT and DALL-E has actually altered how many industries operate as well as how we live our daily lives. These potent instruments are revolutionizing how we use computers, produce content, and resolve challenging issues. These AI systems have a wide range of possible uses, from creating text responses that resemble those of a human to creating beautiful visual art from textual descriptions.
Generative AI will become more and more significant in forming our digital environment as we move forward. Although these technologies present intriguing opportunities, they also present fresh difficulties and moral dilemmas that must be resolved.
FAQ’S
Will human jobs be replaced by generative AI?
Although it’s nearly difficult to forecast the future, generative AI shouldn’t replace occupations; rather, it should improve human abilities and lessen monotonous work, which will make employees’ jobs easier.
What distinguishes generative AI from AI?
In general, computers created to carry out tasks that normally need human intelligence are referred to as artificial intelligence (AI). Generative AI is a subset of AI that specializes in content generation and is distinguished by its capacity to generate unique and innovative content on its own.
How can I enhance the client experience with generative AI?
Generative AI can be used to offer individualized help, produce and update interesting self-service material for your knowledge base, and extract insights from big datasets to enhance your goods and services.